Predicting ASD comorbid Anxiety in Early Childhood via Multi-modality MRI
2025 Award: $59,102
Can the structure of a child’s brain predict their risk for developing anxiety? Our groundbreaking study harnesses the power of deep learning to decode the complex relationship between brain architecture and anxiety vulnerability in children with autism. By analyzing cortical morphometry and white matter microstructure, we aim to identify neuroimaging biomarkers that could revolutionize early detection and intervention strategies. This research bridges a critical knowledge gap in understanding how brain structure relates to anxiety in early childhood autism, potentially transforming how we approach mental health care in neurodevelopmental disorders.
Need/Problem: Research shows changes in brain networks and structure in adults with autism who have anxiety or ADHD, but we know very little about how brain structure relates to anxiety in young children with autism. The high rates of overlap between autism and anxiety makes it extremely difficult to distinguish between symptoms of anxiety and core autism traits.
Grant Summary: We aim to develop a predictive computational model for early identification of anxiety risk in children with autism spectrum disorder. This study will utilize deep learning algorithms to characterize the relationship between cortical morphometric features, white matter microstructure, and anxiety symptomatology. By integrating multimodal neuroimaging biomarkers, our approach offers a comprehensive assessment of structural brain characteristics that may serve as early indicators of anxiety vulnerability in this neurodevelopmental population.
Goals & Projected Outcomes: The goal of this study is to develop novel machine learning methods to predict the individual school-age anxiety scores from early childhood brain structure. We will identify key brain regions associated with elevated anxiety levels, potentially improving targeted interventions. The outcome of this study will provide preliminary results for an NIH grant proposal that will investigate more in-depth individual differences in brain-behavior relationships from early childhood to adolescence.
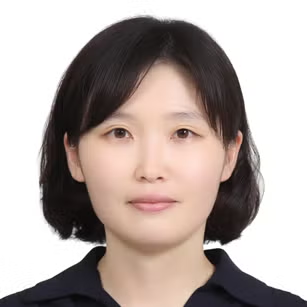
Grant Details: This grant proposes developing and validating a novel model to predict anxiety levels in children using multi-modal MRI data and a graph convolutional neural network (GCN). The GCN model will be developed to predict individual anxiety outcomes from cortical and white matter microstructure, comparing accuracy in subjects with and without comorbid autism. Key brain regions that contribute most significantly to predicting anxiety levels will be identified using explainable AI methods. The primary analysis uses longitudinal MRI data from Infant Brain Imaging Study (IBIS), which tracks infant at high likelihood for autism. The generalizability of the model will be tested using the Adolescent Brain Cognitive Development (ABCD) study dataset, and the GCN model will be enhanced by incorporating an attention-based adaptive adjacency matrix to improve graph topology learning and capture more dynamic inter-node relationships.