Data Driven Brain Shape Analysis Framework and Explainable AI
2022 Award: $66,691
Specific shape aspects are associated with individual differences in cognitive function in children and adults as well as abnormality in many neuropsychiatric disorders. In this project, we will investigate brain shape and reveal specific characteristics for different neuropsychiatric disorders. Our approach is based on multi-view and neural network interpretability techniques.
Need/Problem: The overarching goal of this proposal is to develop deep learning techniques for brain shape analysis. We need to investigate, identify, and implement better approaches that will help us make predictions about health outcomes as well as improve early diagnosis of mental health disorders.
Grant Summary: Brain shape analysis has been researched extensively and we know that specific shape aspects of the White Matter (WM) are associated with individual differences in cognitive function in children and adults as well as abnormality in many neuropsychiatric disorders. Through our shape analysis technique, we will investigate structural and diffusion MRI data. We want to identify specific shape characteristics of neuro-psychiatric disorders to characterize shape in normal patients and compare it to children that are at high risk to explore if/when the group at high-risk group diverges from the normal.
Goals and Projected Outcomes: First, to build a framework for shape analysis of the brain that supports complex shapes such as the white matter surfaces as well as simpler shapes like fiber tracts. Second, a trained model for classification in different psychiatric disorders using fiber tracts as input or cortical surfaces. Third, interpretability mechanism that shows which shape features are important and relevant to the decision of the neural network. Fourth, we will package our tool and distribute it as a standalone application as well as a plugin for 3DSlicer.
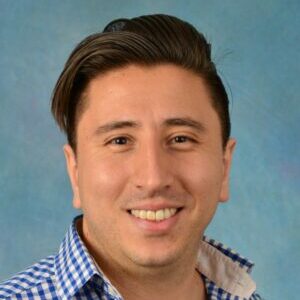
Juan Carlos Prieto, PhD
Grant Details: Shape analysis is a fundamental topic in biology and medicine. Studying shape changes allows us to study growth; how shape changes through evolution; how shape is affected by disease, or changes with other covariates such as sex, age, or environmental conditions. It is common practice to simply quantify the size/volume of structures in neuroimaging studies, however, it is evident that object characterization by this parameter is not enough. Our shape analysis technique uses multiple views from the 3D object and extracts features, learning directly from the 3D data. With our interpretability approach we expect to reveal important shape characteristics and understand the decision-making process of the neural network.