A Machine Learning Approach to Classify Medical Records by Psychiatric Diagnosis
2023 Award: $35,784
The widespread adoption of electronic health records (EHRs) has been accompanied by the enticing promise of using “big data” to improve patient care and clinical research. Many “big data” models rely on discrete data points, while in the field of mental health, our outcomes and diagnostic criteria are typically found in unstructured clinical narratives. In this study, we will train and evaluate machine learning classification models of psychiatric diagnoses using software capable of mining structured and unstructured data in the EHR, by incorporating unstructured clinical data using natural language processing.
Need/Problem: The widespread adoption of electronic health records (EHRs) is accompanied by the enticing promise of using “big data” to improve patient care through large-scale research studies, population health and quality improvement initiatives, and improved clinical decision support tools. While many data informatics tools work well with discrete data elements, in the field of mental health, our outcomes and diagnostic criteria are typically found in unstructured clinical narratives. These unstructured data-rich portions of the EHR present a challenge to machine learning (ML) algorithms that depend on discrete data elements. However, without these tools, mental health researchers and clinical informaticians must devote significant time and resources to inefficiently wade through clinical notes to identify eligible patients for a study or clinical intervention, or to classify cases and controls in a cohort. Similarly, clinical decision tools in the EHR and quality improvement initiatives risk missing important data only available in unstructured text if these tools and initiatives are limited to discrete data elements.
To overcome this challenge and accurately incorporate mental health outcomes into ML models, we must include unstructured data.
Grant Summary: We will train and evaluate ML classification models of psychiatric diagnoses using software capable of mining structured and unstructured data in the EHR. Our models will incorporate unstructured clinical data using natural language processing (NLP). We will first generate a gold standard by manual chart review which will be used to train and evaluate the performance of our models.
Goals and Projected Outcomes: This work will generate a set of trained classification models for psychiatric diagnoses. We will make our results available on the phenotype sharing repository Phenotype Knowledge Base (www.phekb.org). We anticipate building upon and applying the work described in this proposal to broader populations with future collaborators (e.g., investigators through the PheKB repository, such as at Vanderbilt University, or consortia such as PsycheMERGE) with the long term goal of supporting mental health research efforts and providing efficient and effective care to patients with psychiatric diagnoses.
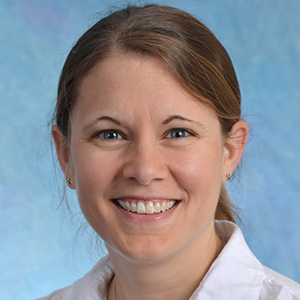
Rebekah Nash, MD, PhD
Grant Details: Data in the electronic health record (EHR) include discrete data elements (e.g., problem lists, lab results, medications) and unstructured data (e.g., narrative text in nursing or physician notes). Machine learning (ML) combines data inputs (e.g., diagnoses or lab results) with algorithms to predict outcomes (e.g., diagnoses), and natural language processing (NLP) extends the reach of these algorithms into unstructured data (e.g., clinician notes). At UNC, in collaboration with CoVar Applied Technologies, the North Carolina Translational and Clinical Sciences Institute (NC TraCS) developed the Clinical Annotation Research Kit (CLARK). CLARK is an ML algorithm that incorporates NLP in a package that is accessible to clinicians. During Aim 1, we will generate gold standard datasets for psychiatric diagnoses by manual chart review of the study cohort (n = 731). During Aim 2, we will train and evaluate classification models for psychiatric diagnoses that leverage both discrete data and NLP of unstructured data by: (a) generating a dataset (corpus) for analysis, (b) training and evaluating performance of classification models against the gold standard for each psychiatric diagnosis, and (c) exploring the impact of text preprocessing and CLARK confidence threshold on classification model performance and compare CLARK model performance to those of other NLP packages.