Developing and Evaluating a Computable Phenotype for Treatment-Resistant Schizophrenia
2021 Award: $41,302
Treatment-Resistant Schizophrenia affects about 30% of Schizophrenia patients. Reliable identification of TRS patients within an Electronic Health Record (EHR) system will improve patient care and enhance clinical research. We will develop a computable phenotyping algorithm by combining several information technologies to characterize TRS patients from an EHR system.
Need/Problem: Treatment-Resistant Schizophrenia (TRS) affects about 30% of schizophrenia patients. However, the utilization rate of clozapine, the only approved antipsychotic for TRS, remains low. Characterization of TRS patients from Electronic Health Records will facilitate early detection of TRS patients and subsequently increase the use of clozapine.
Grant Summary: We will use an array of information technologies (database query, temporal medication mining, and natural language processing) to develop an algorithm that could quickly characterize TRS patients in an Electronic Health Records Systems. The performance of the algorithm will be systematically assessed.
Goals and Projected Outcomes: The goal of the project to generate a computable phenotyping algorithm that could be used to mine Electronic Health Records to identify TRS patients in an automated manner. We will use data from UNC EHR to train and assess the algorithm. We expect the final version of the algorithm to be shared among the computable phenotyping community to be used in other EHR settings.
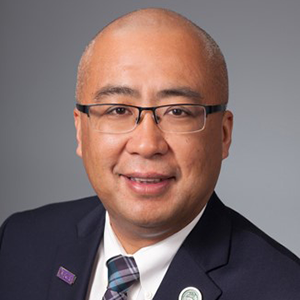
Xiaoming Zeng, PhD
Grant Details: The project is divided into three sequential steps. The first step is to build “gold standard” datasets, during which EHR data will be extracted and manually reviewed by clinicians to label positive and negative TRS cases. Once the datasets are established and verified, we will split them by 70/30 into training and testing datasets, respectively. In step 2, using the training data, we will develop and calibrate the computable phenotype for TRS. We will use combined technologies of database queries, temporal medication mining, and natural language processing to develop and fine-tune the CP for TRS. In the last step, we will evaluate the performance of the CP for TRS. “Gold standard” testing dataset will be used to assess the final performance of CP for TRS. All performance assessments will be measured using standard classification metrics – sensitivity, specificity, recall, precision, Area Under the Curve (AUC), and F-statistics.