Investigation of D2 and 5-HT2A Genetic Variants on Antipsychotic Responsiveness
2025 Award: $57,705
Many patients with schizophrenia endure months or even years of suboptimal treatment before finding an antipsychotic that works. This project aims to change that by identifying how individual genetic differences in brain receptors affect treatment response. The ultimate goal: a personalized tool that helps doctors choose the right antipsychotic the first time.
Need/Problem: Antipsychotics are the cornerstone of schizophrenia treatment, yet patient responses vary widely. Many patients endure a lengthy trial-and-error process during medication selection, which often leads to prolonged symptoms and hospitalization, unnecessary side effects, and poor long-term outcomes. A precision-medicine approach is urgently needed to guide antipsychotic selection using a person’s unique genetic information.
Grant Summary: This study will analyze how genetic variations in two key neuroreceptors—D2 and 5-HT2A—affect a patient’s long-term clinical stability and response to antipsychotic medications in people with schizophrenia. Data collected will support the development of a computational model that recommends optimal antipsychotic choices based on patient genotype.
Goals & Projected Outcomes: The short-term goal is to identify receptor gene variants that predict effective and tolerable antipsychotic regimens. This data will support the creation of a predictive tool for antipsychotic selection and contribute to a future NIH grant application focused on personalized schizophrenia treatment.
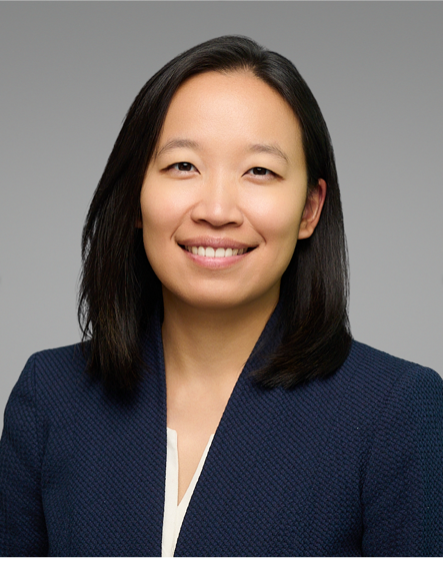
Grant Details: Despite the availability of antipsychotics, most individuals with schizophrenia experience unpredictable responses to medications and poor long-term treatment stability. This project seeks to shift away from the trial-and-error process in selecting an antipsychotic by identifying genetic variants in dopamine (D2) and serotonin (5-HT2A) receptors that influence treatment outcomes. Clinically stable patients will be identified and recruited from the UNC Schizophrenia Treatment and Evaluation Program (STEP). Genetic data on these neuroreceptors will be correlated with patients’ medication history to build a predictive tool for antipsychotic selection. The study’s integrative design combines molecular pharmacology, AI-based protein modeling, and machine learning. Results will form the foundation of a future NIH proposal to expand this personalized medicine approach to broader clinical use.